Predicting Collections Activity
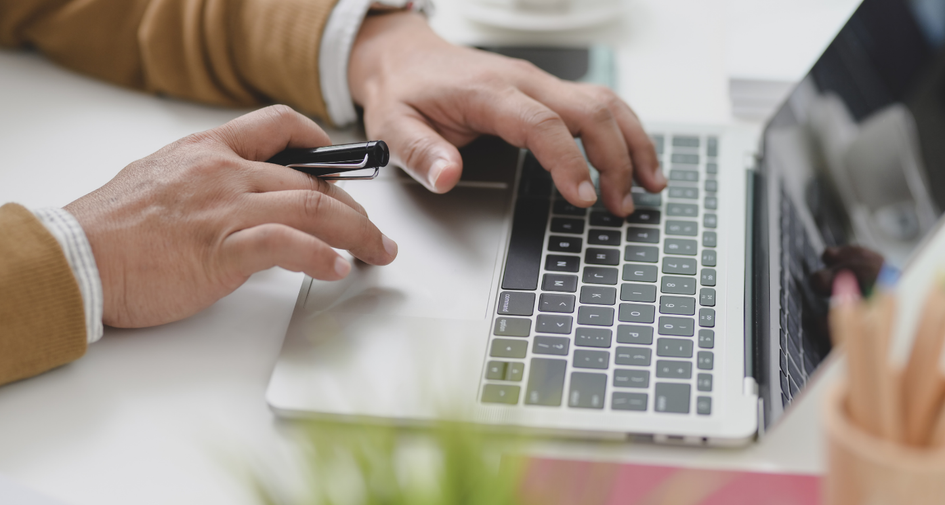
Motivation
There can be large fluctuations from month to month with collections activity, which can lead to many collections leaders scratching their heads as to why they vary so much. A forecast that is wildly off can be detrimental to the overall cash position. Predictability in cash flow is incredibly important and the P(Roll) model developed by Tesorio aims to help companies understand the month-to-month cash fluctuations.
The P(Roll) Model refers to the statistical notation of the Probability of Roll of an individual invoice. We define “Roll” according to the Roll Rate definition used in consumer finance of how many accounts or invoices become more or less delinquent. For example, if an invoice is in the 31-60 day delinquency bucket one month, and the following month is in the 61-90 day bucket, we would consider that invoice to have “rolled forward”. Alternatively, if that invoice moved closer to current or paid in full, this invoice would have “rolled backward.” We can simplify this to calculate the percentage of invoices and the percentage of outstanding dollars collected from month to month.
The P(Roll) model is a model designed to generate the probability of an invoice either becoming more delinquent or receiving a payment. Effectively, the output for a single invoice will consist of two values, with one value being the probability of rolling (more delinquent), and the second value being the probability of receiving payment. Since they are probabilities, these probabilities will always sum to one.
Forecasting Collections
The P(Roll) model is an additional module on top of our cash forecasting model and does a good job of forecasting the dollars collected over the upcoming month. We multiply the probability of payment being received in the period by the remaining invoice balance to calculate the expected value for that invoice over that month. When we compute the sum of the invoice expected values, this derives the forecasted collections for that month.
With all machine learning models, it is important to backtest, or validate, how well the model performs. We do that by looking at the predictions versus actuals.

Figure 1 above shows predictions versus actuals for one-month ahead forecasts. These are based on data across all Tesorio customers, and are equally weighted and normalized to remove any identifiable characteristics. This P(Roll) model is able to predict the collections one month ahead, and closely predict the potentially large fluctuations month-to-month. This approach does a significantly better job of predicting the number of invoices that have rolled or been paid in full than other approaches such as generating a predicted pay date or a time series model approach.
Application in Collections Difficulty
The P(Roll) model fundamentally predicts whether an invoice will be paid in a certain period. An externality of the P(Roll) model is that it can be used as a measure of how difficult an invoice is to collect. An invoice with a high probability of rolling could also be considered as “difficult” to collect.
Typically, the more delinquent an invoice becomes, the lower the likelihood of collections on that invoice being possible. Across our companies, we see declining probabilities as the delinquency bucket becomes further past due. For example, the 31-60 day bucket has lower collection probabilities as a whole than the 1-30 day bucket, which implicitly means that the 31-60 bucket is harder than the 1-30 bucket. Due to each bucket having vastly different probabilities, we scale the probabilities onto a 0-1 scale based around the mean value of that bucket. In this manner, we can show the overall level of difficulty for a specific bucket, as well as identifying how difficult an individual invoice is to collect on.
Implications for Managers & Collectors
This model can be valuable for both A/R Managers and individual collectors. A manager can use this score to evaluate whether an individual collector was lucky or good—the variance between the collected amount and the predicted value can be considered the delta of “skill”. This score can also be used in scenarios with multiple collectors and the manager wants to ensure there is an even load spread among the individuals. As far as an individual collector is concerned, they can use this score to prioritize their outstanding A/R that they are responsible for, choosing to message differently, or take more aggressive actions with difficult invoices, and more quickly working through those that can be expected to perform better.
Conclusion
The P(Roll) model is a highly accurate model predicting the probabilities of an invoice rolling or being paid in full. This model can be used to generate highly accurate forecasts of collections during the upcoming month as well as helping to explain the month-over-month differences in collections amounts. A significant externality of this model is the ability to function as a “collections score” which can be used when evaluating individual performance.
This model is one of many high-performance machine learning models available inside of the Tesorio platform. Please reach out to sales@tesorio.com for more information and to see how we can help you hit your collections goals more predictably.